IFPEN is a global leader in the development of fossil feedstocks hydrotreatinga for clean fuel production. Processes from the same family now apply to a broader diversity of feedstocks: plastic and tire pyrolysis oil, in the context of chemical recycling, vegetable oils for biofuel production, etc. For these processes themselves to be eco-efficientb, beyond the targeted environmental benefit, their operating conditions need to be optimized by the use of kinetic or hybrid modelsc, as a function of the feedstocks employed and the specifications sought for the target products.
In a competitive context, new processes have to be developed fast and it is necessary to minimize the experimental phase, which is always time-consuming and costly, without undermining the quality of the models it feeds into. This is where the TLd approach comes in, consisting in pre-training a model in a similar field and then adapting it to a specific problem so as to take advantage of knowledge already acquired.
At the end of a thesis conducted in partnership with the “Process Experimentation” Division [1], Bayesiane and Monte Carlo Markov Chainf methodologies were implemented to transfer information from the “fossil world”, such as the development of old catalysts, to new catalysts and new processes [2, 3].
This approach produced two main benefits:
- Concerning a new catalyst, a model was trained using old data relating to pilot and industrial facilities. The model was then transferred to the new catalyst and turned out to be of far better quality than that obtained using traditional techniques (RMSEg = 4.8°C for the old method, 2.9°C for the new).
- Concerning new feedstocks, and particularly the Rewind Mixh process for purifying plastic waste pyrolysis oils, a new model was very quickly developed with a reduced number of experimental points (see Figure 1). The transfer learning approach also increases the model’s robustness..
This research has also been extended to more traditional hydrotreatment fields and no fewer than five research projects have benefited to date, to accelerate the availability of new models and the reduction in their access costs.
In the context of the ecological transition and the associated need to treat new feedstocks, either bio-based or recycled, this research is an important contribution to the development of new processes. By building on previous knowledge, it helps accelerate development, representing a significant competitive advantage in these emerging markets.
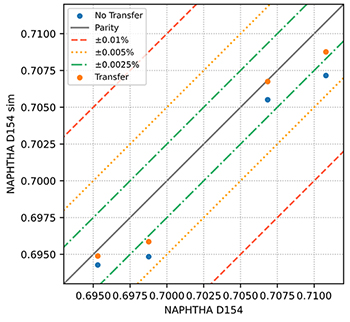
a- This process consists in removing heteroatoms (N, S, etc.) and cracking the molecules to produce fuels. To achieve the required specifications, the use of hydrogen is essential.
b- Eco-efficiency expresses the relationship between economic benefit and the environmental impact caused.
c- Combining traditional chemical approaches and the latest advances in the field of data science.
d- Or transfer learning.
e- Statistical approaches based on Bayesian inference, whereby the probability expresses a degree of belief in an event.
f- https://www.lemonde.fr/blog/binaire/files/2016/06/MCMC.pdf
g- Root mean square error.
h- https://www.axens.net/markets/plastic-recycling
i- Light hydrocarbons recovered from the top of the distillation column
References:
-
L. Iapteff, Apprentissage par transfert pour l’analyse prédictive intelligente, Thèse IFPEN, Université Lyon II, 2022
>> https://theses.fr/2022LYO20007
-
P.J. Becker, L. Iapteff, B. Celse, Improving Model Robustness with Transfer Learning for Product Property Models, Proceedings of the 33rd European Symposium on Computer Aided Process Engineering / 14th International Symposium on Process Systems Engineering (ESCAPE33/PSE23), 2023.
>> https://doi.org/10.1016/B978-0-443-15274-0.50168-2
-
W. Pejpichestakul, P.J. Becker, B. Celse, Transfer Learning of Hydroprocessing Model from Fossil Feedstocks to Waste Plastic Pyrolysis Oil, Proceedings of the 34th European Symposium on Computer Aided Process Engineering / 15th International Symposium on Process Systems Engineering (ESCAPE34/PSE24), 2024.
>> https://view.officeapps.live.com
Scientific contact: benoit.celse@ifpen.fr