One of IFPEN's main areas of research concerns processes and catalysts for the production of bio-based fuels. This is reflected in a large number of experimental projects, the results of which generate knowledge that is then harnessed to develop models. However, this modeling requires the acquisition of large sets of experimental data, which are costly in terms of both time and resources.
Historically, IFPEN has built up an extensive experimental database and developed kinetic models aimed at predicting catalytic activity for the conversion of fossil hydrocarbon feedstocks. To adapt this expertise from petrochemicals to bio-based chemistry, a first thesis was dedicated to transfer learning, using a Bayesian1approach to identify new parameters in existing models [1]. A second thesis [2] is currently underway focusing on other transfer learning methodologies derived from recent data science developments: its aim is to accelerate the development of kinetic models for new generations of catalysts adapted to new bio-based feedstocks.
1 A statistical inference method used to calculate the probabilities of various hypothetical causes from the observation of known events
Two approaches are being studied: model-based and data-based. The first approach is based on an existing model and transfer learning includes the structure of this model, with learning hinged around new data in this case. The second approach combines new data (bio-based feeds) with old data (fossil-based feeds) to make predictions on the new domain.
For both these approaches, of equivalent prediction quality, the number of new data required to learn the new model has been shown to be lower compared to the methodology without transfer.
Both methodologies were applied to synthetic data2 created from kinetic denitrogenation models developed at IFPEN for the hydrocracking process. The results shown in Figure 1 correspond to the predictions made on the target base as a function of the number of points used. Model quality is assessed by calculating the mean absolute error3 of nitrogen content over 20 independent simulations.
2 Data obtained by calculation, representative of field reality (e.g. reaction temperature, pressure, input and output nitrogen composition). Around 50,000 points simulated
3 Mean absolute error on figure 1: represents the difference between the experimental and simulated values
The without transfer scenario, used as a reference, corresponds to the precision we could obtain if a new model is developed solely on the target base. The quality of the model obtained is inadequate, even if 25 points are used. When transfer learning is used (data based), the prediction error is significantly lower for a similar sample size. Moreover, if model-based transfer is used, the mean prediction error is reduced to half. This is due to the addition of knowledge in the form of a kinetic model, which is not the case with the data-only strategy.
This research is contributing to the definition of a methodology that exploits the knowledge acquired in the “fossil domain” to optimize the development time of new bio-based processes, such as the co-processing of vegetable oils.
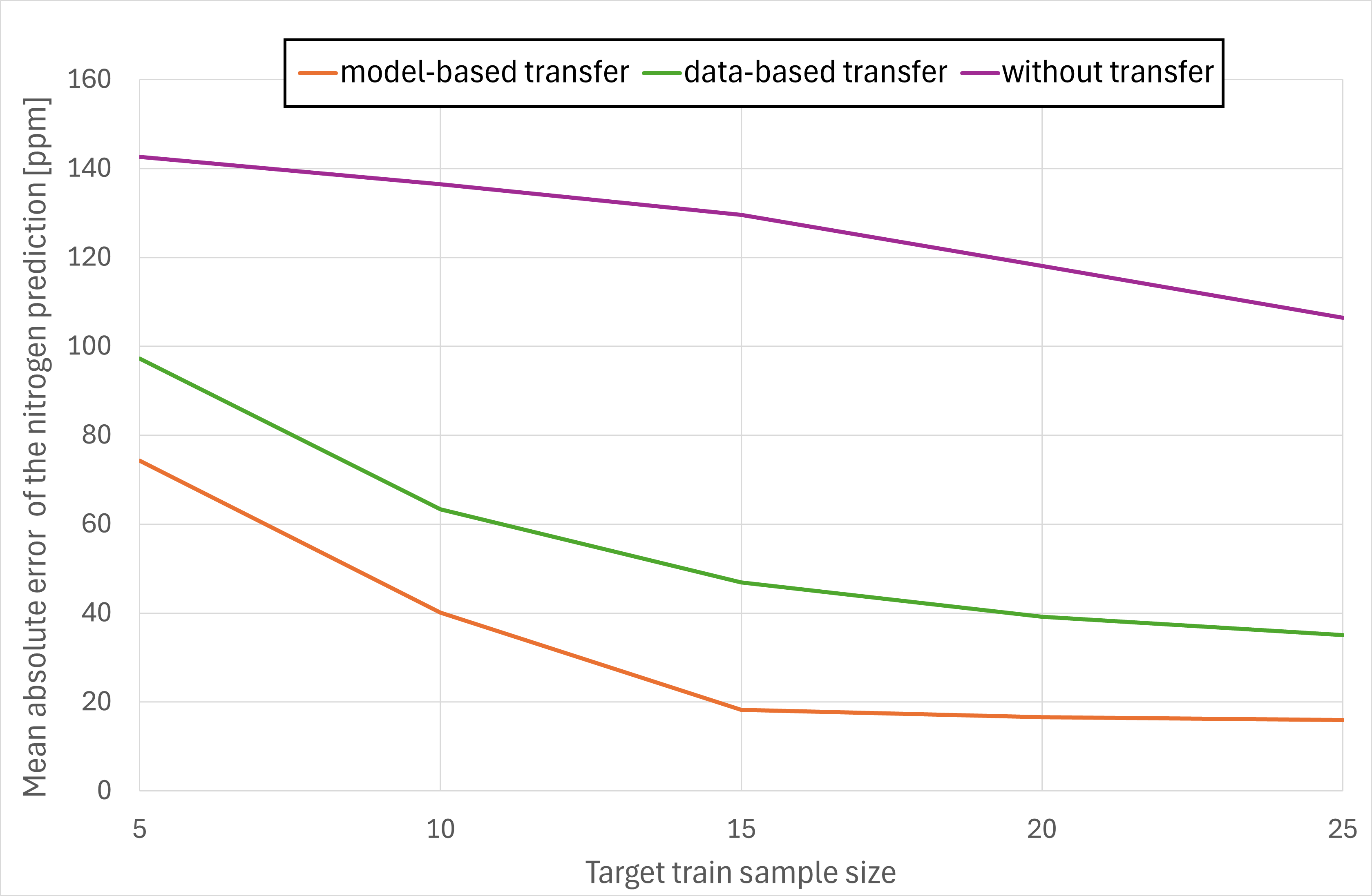
References :
- L. Iapteff, Apprentissage par transfert pour l’analyse prédictive intelligente, Thèse IFPEN, Université Lyon II, 2022
>> DOI : https://theses.fr/2022LYO20007
- Y. Abed, Transfert de modèles cinétiques d’hydroprocessing de charges fossiles à des charges NTE par Transfert Learning, Thèse en cours, Université de Lyon 1, Claude Bernard...
Scientific contact : Victor Costa