The digitalization of activities is, in many respects, a major step forward that IFPEN is committed to rolling out for the benefit of its R&I. It consists in integrating innovative digital technologies to improve the efficiency, precision and productivity of our activities. It also involves integrating promising applications in generative artificial intelligence (AI).
In the field of pilot units, the DataUP project, led by IFPEN, is aimed at structuring and promoting experimental data and making it accessible to all. The process implemented is hinged around three key steps (Figure 1): data preparation, data storage and date exploitation. During preparation, data are collected from various sources and go through a governance step1. The data is then standardized, aggregated and centralized in a data lake, ensuring accessibility and durability. Users can then exploit these data via visualization tools or through personal requests.
1 Data governance is the set of practices implemented to ensure the security, confidentiality, accuracy, availability and usability of data.
There are many benefits to this new approach to data management. Firstly, centralized access to experimental data makes it easier to consult and use. It also makes it possible to make informed decisions, based on reliable, validated data. Monitoring and using results are also made easier by the recourse to visualization tools.
In addition, the introduction of a reference system for magnitudes ensures homogeneity of definitions between the different research teams. Finally, the clear separation between the different tools (for data structuring, visualization and calculation) means that each tool can be updated or enhanced independently of the others, without compromising the system as a whole. Moreover, data are not modified at source, ensuring the integrity of the original data for future use.
Digitalization increases research and innovation capacity. One example of this is provided by the RING software, designed to efficiently manage the workflow for producing experimental data from various chemical engineering processes. It represents a major development compared to pre-existing software, integrating advanced functions for acquiring and using results. As a primary data source, it plays a pivotal role in IFPEN's information system.
RING has three key functionalities: data aggregation, calculation performance and data exploitation.
Aggregation involves sorting and prioritizing the experimental data to be computationally enriched. This enrichment involves verifications, such as material balances, or the generation of performance indicators, such as a conversion rate for a reaction process.
All these calculations are standardized and based on a variety of sources, such as measurements from pilot plant sensors, online or off-site analyses, and catalytic data.
Finally, the data can be used to extract experimental points for multi-criteria research or to produce data for kinetic modeling.
As previously stated, the benefits of setting up RING are the capitalization and long-term preservation of experimental data and associated “profession calculations”, along with simplified access to all these data.
Two main tools are available to users for data visualization. One provides rapid access to data from a variety of sources, facilitating extraction for modeling purposes. Benefits include the identification of relevant formulation and operating conditions, and the search for anomalies. The second tool displays “time series”-type data such as pilot unit sensors or online analysis data. This tool enables pilot units to be monitored on a daily basis and sheds light on incidents.
In addition to exploiting the results of the processes integrated in DataUP, these tools are invaluable aids for comparing and exploiting large-scale tests. Data visualization tools are also useful in areas far beyond their intended scope. For example, an application developed for automated monitoring of utility consumption has resulted in significant time savings for its manager.
Finally, the structuring and availability of experimental data made possible by these developments open up a wide range of avenues, some of which have already been exploited, for the deployment of solutions based on artificial intelligence.
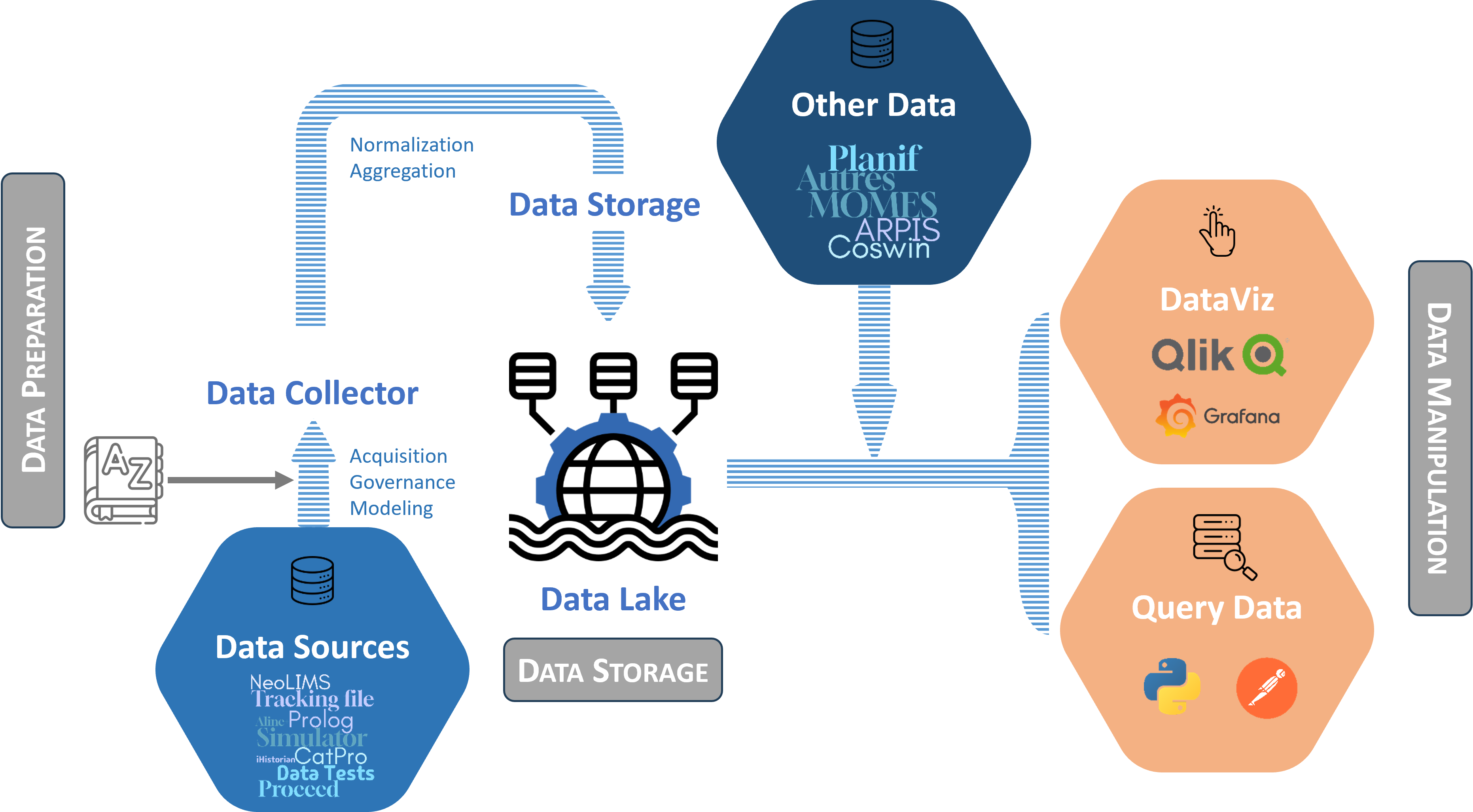
Scientific contact: Alice Faure