Thesis prepared by Loïc Iapteff: "Transfer Learning for Smart Predictive Analytics"
IFPEN is a global leader in the development of catalysts and processes for clean fuel production. For these processes themselves to be eco-efficient1, it is necessary to optimize the coupling of catalysts with the operating conditions, as a function of the feedstocks used and the target specifications for the refined products. It is therefore useful to be able to draw on predictive models for the performance achieved, and machine learning can help improving these models.
For each new process and each new catalyst, the model has to be trained with experimental data acquired on pilot facilities. The operation of these facilities is time-consuming and costly, which is why it is important to drastically reduce the volume of experimental data needed to develop new generations of catalysts, while maintaining the quality of the predictive models. This is where transfer learning comes in, an approach consisting in pre-training a model in a similar field, and then adapting it to a specific problem so as to take advantage of knowledge already acquired.
Bayesian2-type techniques were implemented within the framework of this thesis to transfer different model types [1, 2], the main advantage being the reduction in the number of observations needed to obtain a new efficient modeling process. This is illustrated in Figure 1 relating to the nitrogen content for the hydrocracking pretreatment process: the effectiveness of Bayesian transfer compared with simple data addition can be seen, especially when the volume of data added is low.
This transfer learning methodology made it possible to significantly reduce (by 30%) the number of experimental points needed to optimize models relative to the hydrotreatment process for new generations of catalysts. This research has already been extended to other applications and no fewer than five research projects have from this methodology. This has contributed to accelerate the development of new models while reducing the associated costs.
For the development or improvement of predictive models in the industrial sector, it is necessary to have access to large volumes of data. The current trend is to produce more data. Our work will help to counteract this trend using an innovative and efficient method. With this method, it will be possible to model a phenomenon on the basis of a reduced quantity of experimental data, exploiting prior knowledge to the full.
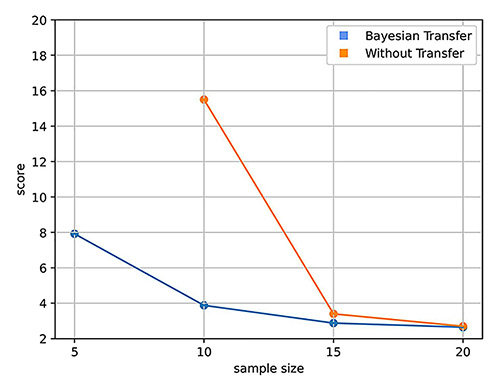
1- Eco-efficiency expresses the relationship between economic benefit and the environmental impact caused.
2- Statistical approaches based on Bayesian inference, whereby the probability expresses a degree of belief in an event.
References
-
L. Iapteff, J. Jacques, M. Rolland, B. Celse, Reducing the Number of Experiments Required for Modelling the Hydrocracking Process with Kriging Through Bayesian Transfer Learning, Journal of the Royal Statistical Society Series C Applied Statistics, 70(5), July 2021
>> DOI: 10.1111/rssc.12516
-
L. Iapteff, J. Jacques, V. Costa, B. Celse, Reducing the Number of Experimental Points to Fit Kinetic Models: A Bayesian Approach, July 2023
>> DOI: 10.1021/acs.iecr.2c03862
Scientific contact: victor.costa@ifpen.fr