Thesis prepared by Adam Hammoumi: "Analysis-Driven Design of Digital Multi-scale Microstructures of Materials"
While macroscopic models combined with experimental analysis of porosity are well established for geometrically simple pores, hierarchized and disordered microstructures defy existing frameworks and call into question conventional interpretations. We proposed a digital framework to help overcome this challenge, taking into account morphology, connectivity and pore size distribution.
On the basis of realistic digital twins, our strategy firstly made it possible to faithfully reproduce the results of porosity characterization techniques [1]. New numerical simulation models also made it possible to associate physicochemical phenomena with the geometric properties of microstructures [2]. Lastly, calculations conducted on the basis of these models were accelerated by a factor of 30 via deep learning (example in figure 1), thanks to our auto-encoding model enabling, for large volumes, precise simulation of mesoporosity down to the nearest nanometer [3].
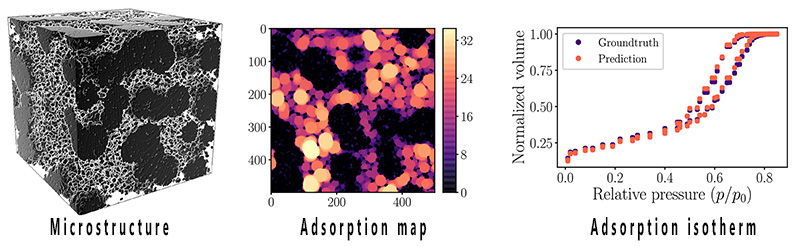
The results of this PhD research are very encouraging and the simulation platform developed also paves the way for virtual tests to reproduce operations on materials, such as grinding, and provide access to a unique vision of the internal transformations undergone by microstructures during them.
Beyond the usual processes of microscopic modeling and the experimental characterization of porous material microstructures, the approach employed is also aimed at establishing a new rapid and adaptive digital framework, with a view to:
- understanding the relationships between the textural and usage properties of complex porous materials;
- being able to obtain a “rapid flow” of simulated experimental results.
[a]- https://www.ifpenergiesnouvelles.com/brief/numerical-design-based-analysis-multi-scale-porous-material-microstructures
[b]- https://www.ifpenergiesnouvelles.com/brief/sc4-new-numerical-approach-characterization-virtual-porous-materials
[c]- https://www.ifpenergiesnouvelles.com/brief/semantic-segmentation-through-deep-learning-materials-sciences
References
- A. Hammoumi, M. Moreaud, D. Jeulin, E. Jolimaitre, T. Chevalier, L. Sorbier, M.Klotz and A. Novikov. A novel physisorption model based on mathematical morphology operators preserving exact pore morphology and connectivity. Microporous and Mesoporous Materials, Volume 337, 2022.
- A. Hammoumi, M. Moreaud, C. Ducottet and S. Desroziers. Adding geodesic information and stochastic patch-wise image prediction for small dataset learning. Neurocomputing, Volume 456, 2021.
- A. Hammoumi, M. Moreaud, E. Jolimaitre, T. Chevalier, A. Novikov and M. Klotz. Efficient Pore Network Extraction Method Based on the Distance Transform. In Lecture Notes in Networks and Systems, vol 144. Springer, Cham. Proceedings of the Artificial Intelligence and Industrial Applications conference (A2IA 2020),
- A. Hammoumi, M. Moreaud, E. Jolimaitre, T. Chevalier, A. Novikov and M. Klotz. Graph-Based M-tortuosity Estimation. In Lecture Notes in Computer Science, vol 12708. Springer, Cham. Proceedings of the Discrete Geometry and Mathematical Morphology conference (DGMM 2021).
- A. Hammoumi, M. Moreaud, E. Jolimaitre, T. Chevalier, A. Novikov and M. Klotz. Accelerating a Morphology-Preserving Adsorption Model by Deep Learning. IEEE International Conference on Image Processing (ICIP 2022).
Scientific contacts: adam.hammoumi@ifpen.fr ; Maxime Moreaud