IFPEN uses numerous fluids in its fields of innovation for a variety of applications, ranging from renewable energy production to sustainable mobility. Many of the fluids are complex mixtures and the chemistry of their components (hydrocarbons, alcohols, esters, etc.) varies depending on the target application: combustion, cooling, lubrication, electrical insulation, etc. Irrespective of the application, it is vital that the products in question retain all their properties over time, hence the crucial importance of their stability. In this respect, degradation as a result of oxidation is one of the phenomena liable to alter the quality of certain fluids and thus reduce the efficiency of the system employing them, sometimes to the point of system failure.
In this context, a research project, launched in partnership with Lille University, is hosting a PhD thesisa focusing on the issue of fluid reactivity. The aim is to identify the key physical and chemical characteristics for predicting their oxidation stability. To do so, an original approach is being used based on the establishment of global models (figure) comparing and combining two supervised machine learning approaches already successfully employed independently at IFPEN:
- Cheminformatics and principally the development of QSPR (Quantitative Structure Property Relationship) models. These multivariate models provide property values using fluid structure and molecular compositions as input data [1];
- Chemometrics and, more specifically, the development of models based on mid-infrared (MIR) spectroscopy or near infrared spectroscopy (NIRS) These multivariate models, often developed based on “components” (PCAb, PLSc), associate properties of interest with vibrational bands characteristic of the fluids analyzed [2].
By analyzing the information extracted from these two types of representation, it will be possible to construct new models. Capable of describing and predicting fluid stability, they will contribute to:
- the development of new formulations to take into account future constraints linked to new usages, and even the identification of new product sectors, within the context of the energy transition;
- the prediction of fluid reactivity in real operating conditions, with a view to proposing improvement solutions.
The idea then is for these models to be implemented in numerical simulation tools, such as the ReFGend thermodynamic property estimation software for “Transport” applications.
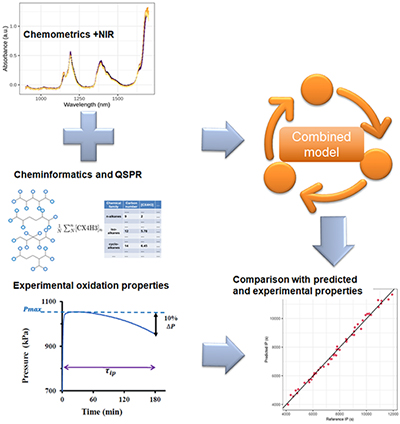
a- Thesis title: “Investigation of descriptors for the understanding and prediction of fluid oxidation stability by via machine learning”, by Adrian Venegas Reynoso
b- Principal component analysis
c- “Partial least squares” method
d- Representative Fuel Generator
References:
- Creton B., Chemoinformatics at IFP Energies nouvelles: Applications in the Fields of Energy, Transport, and Environment. Molecular Informatics 2017;36(10).
>> https://doi.org/10.1002/minf.201700028
- Guillemant J, Albrieux F, Lacoue-Nègre M, Pereira de Oliveira L, Joly J-F, Duponchel L., Chemometric Exploration of APPI(+)-FT-ICR MS Data Sets for a Comprehensive Study of Aromatic Sulfur Compounds in Gas Oils. Analytical Chemistry 2019;91(18):11785–93.
>> https://doi.org/10.1021/acs.analchem.9b02409
Scientific contacts: lucia.giarracca@ifpen.fr ; benoit.creton@ifpen.fr ; marion.lacoue-negre@ifpen.fr
You may also be interested in
Mix between kinetic and machine learning models
Having access to increasingly precise and robust extrapolation models to be able to predict certain propertiesa remains a major challenge for chemical processes industry.
Spectrometry and chemometrics supporting processes
As a result of the deterioration in the quality of crude oils and the tightening up of environmental standards, refiners are modifying their processes in order to meet the growing demand for light