Image analysis is widely used for the microstructural characterization of materials. Digital microscopy images provide representative magnitudes of the texture, which impact global properties (for example diffusion or mechanical).
Semantic segmentation conducted on microscopy images is a processing operation carried out to quantify a material’s porosity and its heterogeneity. It is aimed at classifying every pixel within the image (on the basis of degree of heterogeneity and porosity). However, for some materials (such as aluminas employed for catalysis), it is very difficult or even impossible using a traditional image processing approach, since porosity differences are characterized by small contrasts and complex textural variations.
One way of overcoming this obstacle is to tackle semantic segmentation via deep learning, using a convolutional neural network1. This method, here supervised, requires a reference base built on microstructural images and their hand-segmented equivalents in which the pixels in each zone have been assigned a binary value (0 or 1) corresponding to their degree of porosity (high / low). The creation of this base is extremely laborious and can only be considered for a limited number of images.
During the learning phase, the network learns to convert a gray scale image (8 bit code2) into its binary equivalent, thereby differentiating the forms of heterogeneities present in the microstructure. Learning takes place on reduced zones (patches), both to increase the number of data (several patches per image) and to facilitate learning with a smaller network, comprising fewer parameters to be optimized [1]. This particular way of proceeding, made necessary by the limited size of the learning base, may lead to imprecisions on the edges of the subsections. A sampling strategy stochastically assembling the patches predicted by the network is then used to compensate for this effect (figure).
These new image analysis opportunities based on deep learning, combined with traditional techniques, provide fresh and unprecedented data that can be used to guide the manufacture of porous materials. This segmentation method has been successfully used to characterize different types of alumina catalyst supports [2], after a peptization operation aimed at providing the porous network with a hierarchical organizational structure3.
In the different zones of textural heterogeneity observable using scanning electron microscopy, porosity was quantified using a new measurement method on a local scale [3]. Combined with the hypothesis of a dense barrier formed around the zones of highest porosity, these new results explain the effective differences in diffusion properties macroscopically measured on catalyst supports [2].
Click on the picture to enlarge
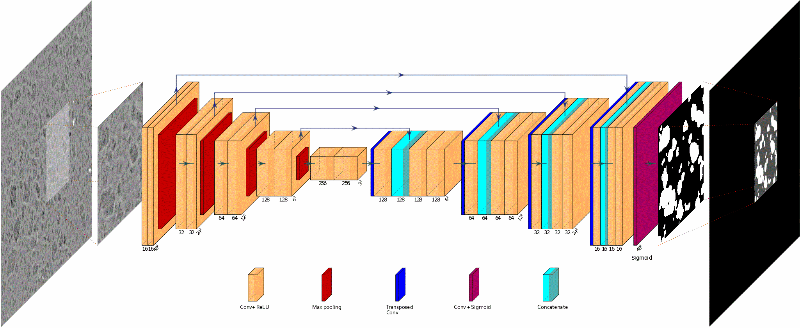
1- Multilayer neural networks with a connection architecture inspired by that of the visual cortex of mammals
2- Value of each pixel between 0 and 255
3- With dimensions ranging from the nanometric to the millimetric scale
Publications:
[1] A. Hammoumi, M. Moreaud, C. Ducottet, S. Desroziers, Adding geodesic information and stochastic patch-wise image prediction for small dataset learning, Neurocomputing, 2021, ISSN 0925-2312
>> https://doi.org/10.1016/j.neucom.2021.01.108
[2] A. Glowska, E. Jolimaître, L. Catita, M. Fleury, T. Chevalier, S. Humbert, A. Hammoumi, M. Moreaud, L. Sorbier, MO. Coppens, Multi-technique characterization of hierarchically organized gamma-alumina catalyst supports, Under preparation, 2021.
[3] L. Sorbier, H. Poncet, V. Lecocq, G. Maillet, M. Moula, V. Le Corre, Local porosity measurement from scanning electron microscopy images in the backscattered electrons mode, Microscopy and Microanalysis, 27(1), 20-27, 2021
>> https://doi.org/10.1017/S1431927620024782
Scientific contacts: Maxime Moreaud and elsa.jolimaitre@ifpen.fr